Spark Structured API Overview
- Jatin Madaan
- Sep 16, 2019
- 3 min read
Structured API are of 3 types :
1 : DataSets.
2 : DataFrames.
3 : SQL table and views .
DataSets and DataFrames (df) are distributed table-like collections with well-defined rows and columns .Each column must have same number of rows as all the other columns (although null can be used to specify absence of a value ) , also each column type must be consistent for every row in the collection .
To Spark df and datasets represents immutable , lazily evaluated plans that specify what operations to apply to data residing at a location to generate some output .Action on df is when actual transformations are performed and results returned .
Schema - A schema defines the column names and types of a df . We can define schema manually (in production this is used as we must be sure what data to process) or read a schema from a data source (generally from first few rows ) , this is also called as schema on read.
Spark is effectively a programming language of it's own. Internally spark uses and engine called catalyst that maintains its own type information through the planning and processing of work . This opens up a wide variety of execution optimisations.
Spark types map directly to different languages API's that spark maintain & their exists a lookup table for each of these in Scala , Java , Python, SQL and R . Even if we use spark's structured API from python the majority of manipulations will operate strictly on spark types and not python types.
eg : below does not perform addition in python , it is actually performing addition purely in spark .
df = spark.range(500).toDF("number")
df.select(df["number"]'+10)
In above addition operations in spark , it will convert and expression written in input language to spark's internal catalyst representation of same type information and then will operate on that internal representation .
DataFrame : To spark (in Scala) df are simply datasets of TypeRow. This "Row" type is spark's internal representation of it's optimised in-memory format for computation . So using df (PySpark) means taking advantage of spark's optimised internal format.
Columns and Row Types : Spark column types are like columns fo table , they can be integer , string , complex - array , map , null values etc. Records of data is a row . Each record in a df must be of type Row().
Declaring a column to be of certain type
eg :
from pyspark.sql.types import *
b = ByteType()
All spark types are converted at run-time . eg : FloatType(), ArrayType() etc .
Structured API Execution
Execution steps :
1 . Write DataFrame/DataSet/SQL code.
2 . If valid code , spark will convert it to a Logical Plan.
3 . Spark transforms this Logical Plan to a Physical Plan (Checks for optimisation along the way).
4 . Spark then executes this Physical Plan (RDD manipulations ) on the cluster.

Logical Planning
It is first phase of execution and it meant to take user code and convert it into logical plan .
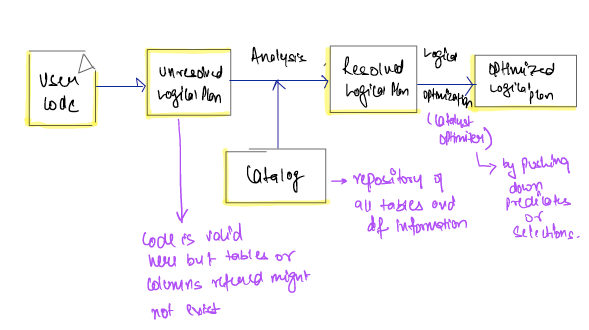
Physical Planning (Spark Plan)
It specifies how logical plan will execute on cluster by generating different physical execution strategies and comparing (choosing how to perform join by looking at physical attributes of a given table , partitions etc ) .
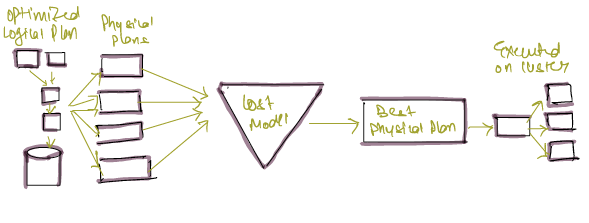
Physical planning results in series of RDD and transformations , this is why sometimes spark is referred as complier as it takes queries in df, datasets & SQL and compiles them into RDD transformations.
Execution
Upon selecting a physical plan , Spark runs the code over RDD's .
Spark performs further optimisation at runtime, Generating native Java byte code that removes entire task or stage during execution.
Results are then returned to user in a file or on CLI .
Comments